A common misconception in statistics is to think that correlation implies causation –
在统计学中,一种常见的误解是认为相关性暗示因果关系——
like, if more tall people have cats, you might think that means being tall makes people more likely to get a cat.
比如有更多高个子的人养猫,你可能会认为这表明长得高更有可能养猫。
However, simply knowing a correlation between height and cat ownership can't tell us which way the causality goes –
但仅仅知道身高和养猫之间的关联并不能告诉我们这种相关性的发展方向——
it may instead be that having a cat causes people to grow taller or perhaps the real cause is something else altogether,
相反可能是养猫导致人们长得更高,或者真正的原因是其他因素,
like that the people and cats live on two separate islands, one a lush paradise with enough food for growing tall and feeding pet cats,
例如,人和猫生活在两个不同的岛屿上,一个是郁郁葱葱的天堂,有足够的食物可以让人长高,还可以喂养宠物猫,
and the other a wasteland that limits both height and cat ownership.
另一个是荒地,限制了身高和养猫。
The point of examples like this is that noticing a correlation between two things doesn't imply that one of those things causes the other.
这几个举例是想说明,两件事情之间的相关性并不能暗示因果起因。
Hence the common refrain: correlation doesn't imply causation. And it's true – it doesn't!
因此,常见的说法是:相关性并不暗示着因果关系。确实如此!
But this often-repeated mantra leads to another common misconception – the idea that you can't infer any causality from statistics. You can!
但这个经常被重复的咒语导致了另一个常见的误解——你不能从统计数据中推断出任何因果关系。是可以的!
I mean, it's quite reasonable to think that, if two things are correlated, there's likely some reason, even if a single correlation can't tell you.
我的意思是,如果两件事是相关的,一定由于某些原因造成是十分合理的,即使单一的相关性不能告诉你这个原因。
Sometimes you can infer the causality from additional information – like knowing that one thing happened before the other –
有时你可以从额外的信息中推断出因果关系——比如事情发生的先后顺序——
but you can also infer causality directly from correlations – you just need more than one, together with something called causal networks.
但是你也可以直接从相关性中推断出因果关系——只不过你需要因果性网络。
Like, in our cat-height-island example, we know that cat ownership and height are correlated,
比如在我们的猫-身高-小岛的例子中,我们知道养猫和身高是相关联的,
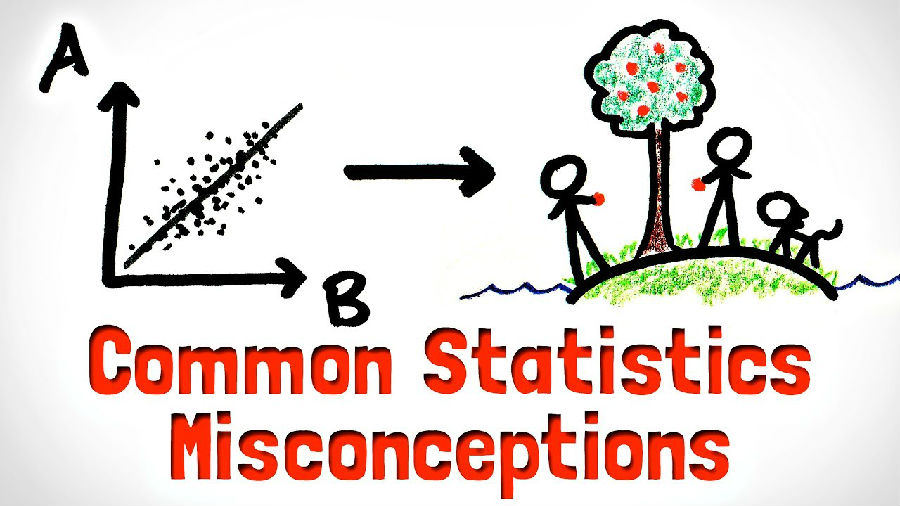
but we don't know what the cause of that correlation is.
但我们不知道这种相关性的起因是什么。
If we don't know anything else, then there are 19 – yes 19! – different causal relationships that could explain the situation.
如果我们不知道其他信息,那么就会有19种——没错,是19种——不同的因果关系可以解释这种情况。
20 if you think the correlation is just an accident. So correlation certainly doesn't imply causation yet.
如果你认为这种相关性只是一个意外,那么就有20种。所以相关性并不意味着因果关系。
However, perhaps we know two other things: first, suppose people born on a particular island stay there,
但或许我们知道其他两件事:第一,假设人们出生在一个特定的岛屿上并常驻,
so their height doesn't influence what island they live on, and we can rule out the relationships where height influences island.
所以他们的身高并不影响他们居住在什么样的岛屿上,并且我们可以排除身高影响岛屿的关系。
Second, suppose that on either island, taken by itself, there isn't any correlation between height and cat ownership;
第二,假设在另一个岛上,并不存在任何身高和养猫之间的相关性;
then we can rule out all the options where height and cats influence each other directly.
那么我们就可以排除所有身高和猫直接相互影响的选项。
This leaves us with just two options: either the islands are the causal explanation for both height and cat ownership
那我们就只剩下两个选项了:要么岛屿是身高和养猫两者的因果解释
(maybe, as before, one island is a lush, healthy paradise for both people and cats),
(或者就像以前一样,一个岛屿对猫和人都是物质丰饶的),
or else cat ownership is the causal explanation for the islands which are the causal explanation for height,
要么养猫是岛屿的原因,而岛屿又是身高的原因,
(like, maybe an abundance of cats turned the island into a paradise, thereby influencing the height of future cat owners).
(比如,大量猫的存在将岛屿变成了天堂,因此影响了未来猫主人的身高)。
So, starting with 19 possible causal relationships, we used correlations to narrow things down to just 2 options – not bad!
所以从19种可能的因果关系开始,我们用相关性把事情缩小到只有两种选择——还不错!
and if we knew something about the time line of when cats and people arrived at the islands
如果我们知道猫和人到达岛屿的时间线,
we might be able to narrow down to just one option.
我们或许能够将选项缩小至一个。
Of course, this is just a simple example, but for any group of things, you can use the various correlations between them
当然,这只是一个简单的举例,但对于任何一组东西中,都可以用它们之间的各种相关性
(or lack of correlations) to eliminate some of the possible cause-and-effect relationships. And that's how correlations can imply causation.
(或相关性的缺乏)来排除一些可能的因果关系。并且这就是相关性如何可以按时因果的。
There is one problem, though, some experiments in quantum mechanics have correlations that rule out all possible cause and effect relationships.
但有一个问题,在量子力学的一些实验中,相关性排除了所有可能的因果关系。
We'll have to save the details for a later video, but until then, here's what that saying really should be:
其中细节,我们下期视频再讲,但现在我们应该这么说:
"Correlation doesn't necessarily imply causation, but it can if you analyze it with causal models. Except maybe not in quantum mechanics."
“相关性并不一定暗示因果,但如果你用因果模型进行分析就可以。除了量子力学问题。”